What is this? MisDisMal-Information (Misinformation, Disinformation and Malinformation) aims to track information disorder and the information ecosystem largely from an Indian perspective. It will also look at some global campaigns and research.
What this is not? A fact-check newsletter. There are organisations like Altnews, Boomlive, etc., who already do some great work. It may feature some of their fact-checks periodically.
Welcome to Edition 43 of MisDisMal-Information
The influence of Influence
This was prompted by recent investigations on influence operations from India (DFRLabs -covered in 42) and Pakistan (Graphika Labs).
I’ve divided the section into 3 parts:
The first part is adapted from my contribution to Technopolitik and delves into how we perceive the effectiveness of influence operations.
Parts 2 and 3 are based on analyses by the Partnership for Countering Influence Operations, which catalogued both how platform policies approach them and the various interventions they’ve enacted.
How influential are influence operations?
In its May 2021 Coordinated Inauthentic Behaviour Report, Facebook disclosed that it had taken down a network that originated in Pakistan and targeted domestic audiences in Pakistan as well as global audiences with content in English, Arabic and Pashto. An accompanying report by Graphika Labs identified 5 kinds of narratives, one of which consisted of content that was ‘anti-India’.
Across the network’s various assets/accounts on Facebook and Instagram, it had ~800,000 followers across 40 accounts and 25 pages, 1200 Facebook group members across 6 groups, and 2400 followers across 28 Instagram accounts. While interesting, these numbers don’t tell us much about how effective the activities of such networks are. Commenting on the era of disinformation operations since the 2010s in Active Measures, Thomas Rid categorised them as more active, less measured, high tempo, disjointed, low-skilled and remote. In contrast, he said, earlier generations were slow-moving, highly skilled, labour intensive and close-range [Aside: It is worth clarifying that Graphika Labs attributed the network to a Public Relations firm and not to a state actor].
Coming back to the question of effectiveness, in Hype Machine, Sinan Aral refers to the concept of “lift” or the change in behaviour caused by a message/series of messages. The word ‘change’ is crucial since it implies the necessity of determining causality, not just establishing correlation. Even more so, when it comes to voting behaviours, political opinions, etc. For this reason, assessments based just on the number of impressions, followers or engagement are incomplete and ignore the ‘selection effect’ of targeting messages to a user who was predisposed towards a certain course of action already. And while lift has not yet been quantified in the context of influence operations due to the complexities of reconciling offline behavioural change with online information consumption, Aral suggests such targeting is most effective when directed towards ‘new and infrequent’ recipients. Or in the electoral context, at undecided voters or those unfamiliar with a certain political issue. In other words, ‘change happens at the margins’.
At least some operations appear to be adapting. Facebook highlights a shift from ‘wholesale’ (high volume operations that broadcast messages at a large scale) to ‘retail’ (fewer assets to focus on a narrow set of targets) operations in a report on the State of Influence Operations 2017-2020. We should expect to see both kinds of operations by different actors based on their capabilities. The thing to note, though, is that there appears to be, at some level, a convergence with an earlier era of disinformation operations.
Postscript: I should add that even though the impression based method does not exclude ‘selection effect’, we don’t seem to have a good way to measure the long term effects of repeated exposure yet. Yes, we do know of ‘illusory truth effect’ (repeated statements are perceived to be more truthful than new statements) and ‘continued influence effect’ (belief in false information persists even after underlying information is corrected/fact-checked) from a fact-checking perspective (and I would argue, from the lens of an individual). What is the effect on this self-selected cohort? And what are the knock-on effects in the context of polarisation (affective and knowledge-based)?
How do platforms approach them?
In April, Jon Bateman, Natalie Thompson and Victoria Smith analysed how various platforms approach Influence Operations based on their Community Standards.
Broady, they concluded, there are 2 types of approaches:
Generalised approaches include the use of short, sweeping language to describe prohibited activity, which enables platforms to exercise discretion.
Particularised approaches include the use of many distinct and detailed policies for specific types of prohibited activity, which provides greater clarity and predictability.
These approaches have implications that go beyond the mere framing of Community Standards.
Generalised
- Based on standards: Loose guides that may require significant judgement
- Give platforms flexibility to enforce in spirit
- Take less time and effort to craft, tweak (potentially better for smaller, newer platforms)
Particularised
- Based on rules: Specified set of necessary and sufficient conditions leading to defined outcomes
- More transparent and predictable for users.
- Can help defuse perceptions or arbitrary decision-making.
Of course, no platform falls completely in either of these buckets, but Facebook and Twitter tend towards the ‘particularised model’.
There’s also a tendency not to use commonly used terms like misinformation, disinformation, influence operations, etc. Instead, the approach has been to try and break them down into sub-categories such as spam, harassment, etc. And, rely on generalised terms and/or self-coined terms like Coordinated Inauthentic Behaviour (Facebook), Coordinated Harmful Activity (Twitter), I-C-BATMAN?NOPE (Damn, I thought I could slip that one past you), etc.
The section that I was most fascinated with was the one about the various elements of platform policies and the ABCDE framework.
A - Actors
B - Behaviours
C - Content
D - Distribution
E - Effects.
Aside: You might recall Camille Francois’ ABC framework from Edition 1 or the ABC(D) modification suggested by Alexandre Alaphilippe in the context of disinformation. The E appears to have gained currency since COVID-19 and (possibly) the 2020 U.S. Elections.
While much of the public conversation is focused on ‘actors’ (content too, to be honest), the policies focus the most on behaviours.
How ‘influential’ are their responses?
I should point out that this sub-heading should include the work effective instead of influential, but I wanted to throw in some wordplay. The other important question is what kind of responses platforms end up formulating and how they actually fare.
Kamya Yadav wrote about this in January 2021.
83 of 92 platform announcements regarding interventions happened in 2019 (17) and 2020 (66). Potential reasons include:
Real-world events (COVID, US elections) could have triggered a new wave of influence operations.
More demands for accountability from governments, media, users, etc.
Malicious actors evolve, necessitating new countermeasures.
Experts have learned more about how influence ops work, resulting in new interventions.
Redirection (53) and Labelling (24) accounted for 77 of the 104 interventions identified. There are also some astute observations about the growing prevalence of these kinds of interventions.
They counter-balance ‘wholesale’ bans and takedowns.
Conversely, they place a greater burden on individual actors to choose how to respond. This is not a value-judgement since this also implies that users have more choice compared with takedowns/bans.
Also notable that most of these were user interface/user experience tweaks. I couldn’t confirm whether the ‘nicer’ News Feed changes that were reversed in December 2020 were considered or not.
Only 8% of the initial announcements stated whether or not the various interventions had been tested for effectiveness before a mass rollout.
Internal Influences
Ok, let’s zoom out a little bit. There’s another aspect of this we should consider - how can it impact domestic politics?
Imagine hypothetical states A and B that have an adversarial relationship. Party X is in power in State A. Domestic opponents in State A criticise/oppose a number of Party X’s actions. State B opposes and criticises a certain subset of these (relevant to state B). Also note, Domestic opponents interest in Party X is significantly higher than State B unless some form of overt aggression from State B is in the picture. Thus, it is inevitable that there will be some convergence between the issues, arguments and narratives employed by Domestic opponents and State B. For simplicity, I haven’t represented internal dynamics within State B.
This presents 2 challenges for Domestic opponents in State A:
Avoid being co-opted/misused by State B operatives.
Avoid being characterised as State B agents or ‘speaking the same language’ by Party X and its allies.
This is not necessarily unique to the Information Age. However, it does present new opportunities for State B to co-opt and misuse legitimate arguments raised by Domestic opponents - which also widens the scope of issues it can use beyond just those that are directly relevant to State B. This was one of the trends Facebook highlighted in its State of IO report:
Blurring of the lines between authentic public debate and manipulation: Both foreign and domestic campaigns attempt to mimic authentic voices and co-opt real people into amplifying their operations.
And, it also gives Party X allies additional opportunities to delegitimise/stigmatise Domestic opponents. Remember, ‘there are no internal affairs’ affects Domestic opponents too.
… Meanwhile in India
▶️ It has been hard to miss the spate of ‘legal request’ notifications that Twitter has been sending various users (just search for mentions of @TwitterIndia). Pranesh Prakash points out that this been in practice ‘for long’. Twitter’s transparency reports for India (available till Jan-Jun 2020 as of writing this) certainly indicates an upward trajectory. We’ll have to wait till January 2022 to see similar numbers for Jan-Jun 2021. Jul-Dec 2021 should be coming out soon, so it will be interesting to see if the upward trajectory holds.
Related:
Twitter restricts accounts in India to comply with government legal request [Manish Singh - TechCrunch]
If you head over to Right Wing Twitter, you’ll chance upon similar screenshots of emails from Twitter due to ‘legal requests’ doing the rounds.
▶️ Ayushman Kaul’s investigation [DFRLab] into Facebook pages operated by Hindu Janjagruti Samiti and Sanatan Sanstha.
Facebook comprised of at least 46 pages and 75 groups to promote hostile narratives targeting the country’s religious minority populations. Leveraging a potential reach of as many as 9.8 million Facebook users, the organization has published written posts, professionally edited graphics, and video clippings from right-wing and state-affiliated media outlets to demonize India’s religious minorities and stoke fear and misperception among India’s majority Hindu community.
As you read this, keep in mind 2 (potentially contradictory) things from the first part of section 1 - the impressions/reach may not directly correlate with effects, and we don’t yet have a way to measure the long-term impact of repeated messaging, even if they are already pre-disposed/have bought into the message(s).
Another report on these groups indicated that Facebook ‘quietly banned’ the pages in September 2020. It points that this was partial, and an additional ‘32 pages with more than 2.7 million followers between them remained active on Facebook until April.’ [Billy Perrigo - Time]
Ayushman’s investigation includes a press release published in Sanatan Prabhat indicating that 4 pages of Sanatan Sanstha were ‘closed’.
Aside: If anyone has figured out how Facebook defines the ‘militarised’ in the context of militarised social movements, please let me know.

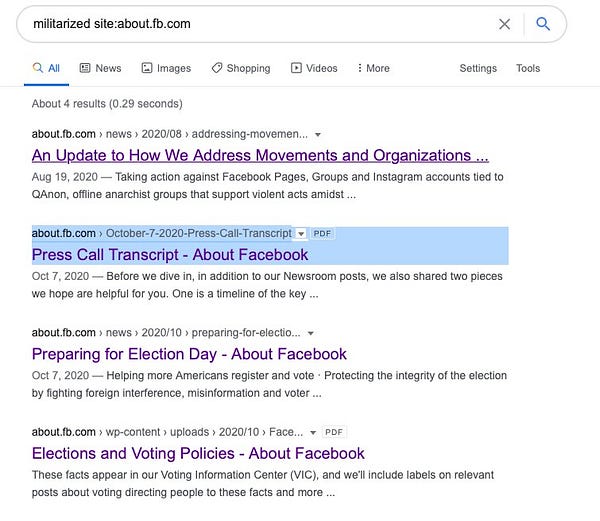

▶️ TeamSaath’s Twitter account was suspended for a few hours on Friday, 11th June. The account, which as its profile suggests stands against "Abuse Troll Harassment", highlights abusive Twitter accounts and encourages users to report them. Now, Twitter does not seem to have an explicit policy against encouraging mass reporting. It is also unknown if the account’s suspension was itself a result of mass reporting 🤷♂️.
Aside #1: From a Katie Notopoulos post in 2017 [BuzzFeedNews] on her experience with being mass-reported.
But for now, Twitter is getting played. They’re trying to crack down on the worst of Twitter by applying the rules to everyone, seemingly without much context. But by doing that, they’re allowing those in bad faith to use Twitter’s reporting system and tools against those operating in good faith. Twitter’s current system relies on a level playing field. But as anyone who understands the internet knows all too well, the trolls are always one step ahead.
Aside #2: In August 2020, Facebook did suspend a network from Pakistan that encouraged mass reporting through a browser extension (they called it ‘coordinated reporting of content and people’).
Ok, getting back. This is where the part about policies focusing heavily on behaviours (from part 3 of the first section) came back to me.
You’ll see that Actors were addressed the least in policies - so it could be that TeamSaath’s stated motivations mattered less. I’ll repeat here, we don’t know why Twitter initially suspended the account.
There’s a growing conversation about context-specific enforcement [Jordan Wildon - Logically]. While I completely understand the motivation behind that approach, I am sceptical of platforms’ abilities to get this right and worry about the type of outcomes it will actually lead to. For example, here’s a random sample of Tweets that were marked ‘sensitive’, and I have not understood why.
Please excuse the shoddy image editing.
#1
#2
#3