Of Participatory Dysfunction, CoMo Infinity and Beyond, Right to Amplify
The Information Ecologist 53
What is this? Surprise, surprise. This publication no longer goes by the name MisDisMal-Information. After 52 editions (and the 52nd edition, which was centred around the theme of expanding beyond the true/false frame), it felt like it was time the name reflected that vision, too.
The Information Ecologist (About page) will try to explore the dynamics of the Information Ecosystem (mainly) from the perspective of Technology Policy in India (but not always). It will look at topics and themes such as misinformation, disinformation, performative politics, professional incentives, speech and more.
Welcome to The Information Ecologist 53
Yes, I’ve addressed this image in the new about page.
In this edition:
Participatory Dysfunction: A paper about a blueprint for cross-platform participatory mechanisms to amplify pro-BJP messages before elections and a race to the bottom as more parties look to adopt it.
CoMo Infinity and Beyond: How much content moderation is too much content moderation?
Right to Amplify: Twitter study about amplification of right-leaning political content on algorithmic v/s reverse-chronological timelines.
Post-publication Updates
18th November 2021: Included a clarification from Mor Naaman in the Participatory Dysfunction section
Participatory Dysfunction
Maurice Jakesch, Kiran Garimella, Dean Eckles, and Mor Naaman recently published a paper about coordinated efforts across platforms to manipulate Twitter’s trends before the 2019 general elections in India [ACM Digital Library].
From the abstract:
We joined 600 WhatsApp groups that support the Bharatiya Janata Party, the right- wing party that won the general election, to investigate these campaigns. We found evidence of 75 hashtag manipulation campaigns in the form of mobilization messages with lists of pre-written tweets.
What are trend alerts?
These trend alerts messages call on group members to participate in targeted mass-postings on Twitter. The messages contain a link to a Google Doc where participants and a list of pre-written tweets to post at a specified time.
Now, this cross-platform nature isn't surprising by itself. Way back in 2019, Pratik Sinha (co-founder of AltNews) had managed to get his hands on, and then edit one such Google Doc. [NDTV]. And Anand Venkatanarayan pointed this out to me as well.

In his book, co-written with Shivam Shankar Singh, 'The Art of Conjuring Alternating', they write about the ON3C framework in the context of hate-campaigns directed at interfaith marriages in India (Objective - Narrative - Context - Campaign - Content). But it was the next chapter about Information Silos that got my attention. They describe 4 steps for dominating the 'information environment' (I'm heavily summarising and paraphrasing here)
Step 1: Conjuring an Information Silo through dismissing/suppressing information opposing / countering a message and promoting those that favour it.
Step 2: Integrating into it embedding themselves in groups and seeding information.
Step 3: Unifying these distinct silos by implanting certain values into them.
Step 4: Nurturing the environment by keeping the audience engaged through 'novel' messaging and keeping contradictory messages out.
Going back to the paper, I was initially drawn to it because of the attribution claim.
For 42 of the 75 investigated campaigns, the tweets we found in the tweet banks could also be posted through the volunteer section of the official Narendra Modi app. This connection proves the BJP’s involvement in the campaigns and shows the extent to which hybrid manipulation campaigns cross the boundaries of platforms and organizations.
But what really got my attention was the section on contribution, implying that at least some of this dysfunction is participatory.
There was a core set of supporters:
The three percent of participants who participated in more than ve campaigns posted 21% of the pre-written tweets.
And what seemed like a rotating cast:
Most supporters joined for only one or two hashtag manipulation campaigns, suggesting loose support driven by occasion and convenience rather than systematic involvement. Paid supporters and bot accounts would likely participate in more than one or two campaigns. Instead, trend alert campaigns are the work of a diverse and disparate group of online supporters.
Aside: I want to caveat the payment part because the authors do say elsewhere that they found no evidence of rewards or payments. It could also be that payment mechanisms are already established and not referenced on the subset of groups of studied.
Update: 18th November 2021
Mor Naaman reached out to clarify some of the phrasing around participation patterns. I am adding it here 👇

End of Update
They also contrast these participatory campaigns from your typical astroturfing campaigns since these were collaborative and voluntary. The observation that activity increased in the run up to various elections (General or state) and dropped off for the periods in between means that such once such systems will be used for various purposes once they are put in place. In fact, Step 4 referenced above implies that they will need to seeded with purpose and content periodically to keep the lines open.
And, the chilling bit i.e. more participatory dysfunction... across party lines.
In our data, we anecdotally observed other Indian parties trying to replicate the method. We believe that trend alerts may have provided a blueprint for participatory media manipulation by political parties with popular support.
Again, I should point out this not surprising. I did write about the use of social media during campaigns in 35: DOOM and The Craft World of War Rooms.
Aside: The term participatory dysfunction is a combination of Kate Starbird’s Participatory Disinformation (39:Of Polarisation, propaganda, backfire and participatory disinformation) and Information Dysfunction (which I realised I referenced but did not explain in 34: Of Hex, Lies and (not just)Video deepfakes, so here’s an interview where Whitney Phillips mentions it). I’ve done this because, even though the participatory nature is evident - I am not sure if all the content was ‘disinformation’ in the sense of false + intended harm, since the participants could actually believe it.
That it is not surprising doesn't it make it any less disturbing for those of who do not want to see a race to the bottom on this front. A series based on Sophie Zhang's Facebook revelations by The Guarding in April 2021, mentions a network that was on Facebook’s radar for engaging in 'Coordinating Inauthentic Behaviour' seemingly switched loyalties (emphasis added). I'm being liberal with the term ‘loyalty’, but you get my point. [Julia Carrie Wong, Hannah Ellis-Petersen - The Guardian]
While Zhang was trying and failing to convince Facebook to take action on the MP’s network, Facebook’s staff took repeated action against one of the two Indian National Congress networks that it had tried to remove in December. Though the checkpoints had knocked out most of the fake accounts, Facebook saw immediate efforts to reconstitute with new accounts and, in the weeks ahead of the 2020 state elections in Delhi, the network that had previously boosted a Congress politician in Punjab began supporting AAP, the anti-corruption party in Delhi.
Aside: See Sophie Zhang's interview with Scroll [Karishma Mehrotra - Scroll.in]
Some more noteworthy observations from the paper:
The authors found no evidence of hate speech against minority groups in the tweet banks that they got access to.
69 of the 75 campaigns made it to the India-wide trending lists.
For many of them, it took about 5000 tweets within 30 minutes to get into the trending lists (it wasn't clear to me if this was for the India-wide lists or the more regional ones)
CoMo to Infinity and Beyond
Just before an extremely interesting talk on 'The Administrative State of Content Moderation', Evelyn Douek included a projection of how many content moderation decisions major Digital Communication Network operators would likely end up taking during the talk. [YouTube]

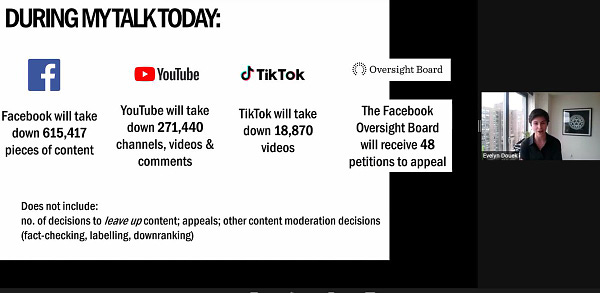
Daphne Keller was kind enough to tweet it out (and that's how I came across it), but just in case the embedded tweet is too tiny, here's a larger screenshot.
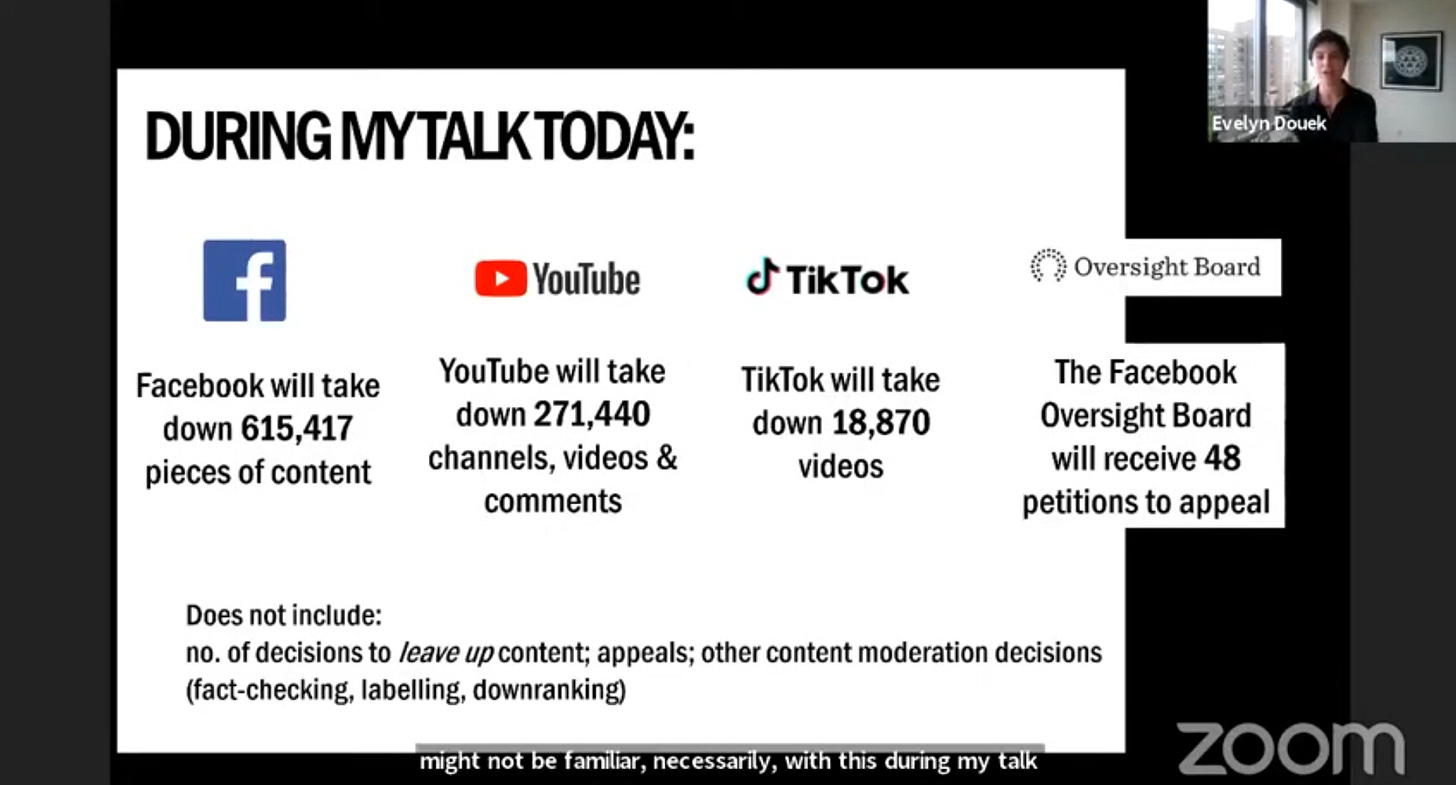
In a post back in September, Internet Freedom Foundation rounded up some of the numbers from the monthly India compliance numbers [InternetFreedom.in]
Aside: It seems infuriating that these aren't accessible unless you have the specific file URLs. Only Google seems to have a page where you can look them up.
Let's look at the proactive monitoring numbers (and just for the sake of context, try to bring them down to average hourly numbers).
Facebook actioned content (which includes removal of content with a warning) relating to 10 issues, viz., adult nudity and sexual activity (26 lakh), bullying and harassment (123.4K), organised hate (94.5K), terrorist propaganda (121.2K), hate speech (324.3K), drugs (30.7K), firearms (4.6K), suicide and self-injury (945.6K), spam (2.56 crore) and violent and graphic content (35 lakh). These figures include proactive action. In each of these categories (except for hate speech, bullying and harassment, and firearms) more than 99% of the content was removed as a result of proactive action by Facebook by using its machine learning technology to detect such content.
Average hourly numbers would fall in a range from 6 / hour (firearms) to spam (36000 / hour). And a total of 42000 per hour (note how spam accounts for ~85%). Facebook also said over 99% were proactive for all but 3 categories (Firearms: 6 / hour, Hate Speech: 450 / hour, bullying: 171 / hour). Even if we exclude those ~ 630 as unknowns, that's still over 99% of the remaining ~41000 as proactive decisions i.e. ~ 40500 / hour.
26,250 accounts are reported to have been suspended due to issues of child sexual exploitation, non-consensual nudity, and similar content. 5,387 accounts were suspended on account of promotion of terrorism.
Based on the data we have, that's around ~ 44 decisions per hour on average.
WhatsApp states that abuse detection operates at three stages of an account’s lifestyle - at registration, during messaging, and in response to negative feedback, which WhatsApp receives in the form of user reports and blocks. ‘Edge cases’ are analysed by a team of analysts which helps improve the effectiveness of the system over time. Indian accounts are identified by a ‘+91’ phone number. Using this abuse detection approach, 30,27,000 accounts were banned by WhatsApp in the relevant period.
Using the same logic, this translates to ~ 420 decisions per hour.
(Which does not differentiate between different properties such as search and YouTube)
Google claims to use automated detection processes for some of its products to prevent the dissemination of harmful content such as child sexual abuse material and violent extremist content. 576,982 removal actions have been taken as a result of automated detection as per the report.
~ 801 decisions per hour
Even though these aren't like-for-like with the numbers Evelyn Douek put up, it seems one can make the case that Facebook, Twitter and Google aren't moderating enough individual pieces of content in India. And yes, that was the point of some of the reporting around the Facebook Files / Papers / Documents anyway.
But, and this is a qualified but, those reports + cases in front of the oversight board also tell us how much Facebook (and I'd say it is extendable to others too) gets wrong through false positives, not just in non-English languages but even in English itself. Not to mention that we're also ceding more political power to these companies, when that's the opposite of what many of us want to do.
Honestly, it is hard to make the case for this, given the amount of hateful/harmful content we see. But I am not sure scaling up these processes to reach infinity and beyond is the answer. I also doubt that we can prepare for explosion in decision making this will require (See question 4) [SochMuch].
Here's where I want to recommend an extremely insightful episode of the Internet of Humans podcast featuring Rebecca Mackinnon where she describes the 100% safety targeting, "fix the internet" regulatory efforts around the world as unrealistic and unattainable (adjectives are my interpretation, listen to the podcast for her views - you won't regret it). [Jillian York and Konstantinos Komaitis - Anchor.fm]
Also, in Evelyn Douek's talk she contrasts the current approach of content moderation (described as an aggregation of individual content moderation decisions) with the need for a forward looking, systemic approach. I don't think we know what that looks like yet, but I think it is worth exploring because, as she says, we'll never agree 100% on individual content moderation decisions, but lets build the systems where we can disagree now (again, I've paraphrased, but this is the point in the video where she says it).
Ad break (no private information was used in targeting this ad)
If you are interested in the topics I write about, then you may want to look at Takshashila’s 12-week public policy courses.
Right to Amplify
Back in October, amid all the noise about the Facebook Files/Papers (should we just call them Documents?) a rather interesting study published by Twitter didn't seem like it got the attention it deserved.
In April 2021, Twitter did say it was going to research the impact of 'ML Decisions' [Twitter.com]
Researching and understanding the impact of ML decisions. We’re conducting in-depth analysis and studies to assess the existence of potential harms in the algorithms we use. Here are some analyses you will have access to in the upcoming months:
A gender and racial bias analysis of our image cropping (saliency) algorithm
A fairness assessment of our Home timeline recommendations across racial subgroups
An analysis of content recommendations for different political ideologies across seven countries
The first of these came out in May 2021. I am not sure if the second one is out yet. But the third was published in October and one I was very interested in [Twitter.com].
They put 9.3 mDAUs (monetisable daily average users) across 7 countries (US, UK, Japan, France, Germany, Spain, Canada) into 2 groups and compared the amplification of political content on the algorithmic (Home) and reverse-chronological (Latest Tweets) from April to August 2021. 80% of these saw algorithmic timelines based on Twitter's personalisation (treatment group) and 20% had the reverse-chronological timeline. It doesn't have a direct India angle, but a study of this nature is worth exploring. For India-related Twitter analysis I would recommend reading a whole bunch of papers co-authored by Joyojeet Pal [ACM Digital Library]
Two limitations that the authors of (Ferenc Huszár, Sofia Ira Ktena, Conor O’Brien, Luca Belli, Andrew Schlaikjera and Moritz Hardt) the study pointed out were:
The control group does exist not in a vacuum and the content they see will be influenced by other users who use personalised algorithmic timelines.
Differences between the control and treatment groups were previously used to inform changes to the 'personalised ranking experience'.
There were 2 parts to the study.
First, based on content posted by ~3600 politicians across all seven countries.
In 6 of 7 countries, i.e. all but Germany, in aggregate, parties on the right were amplified more. It was the most in UK and Canada. In both countries, the conservative parties were in power. However, this didn't change much after removing PM and some prominent ministers.
The Economist claims to have run the data through another model but still found it favoured conservative parties [The Economist]
This discrepancy could arise for reasons besides ideology. To test alternatives, we fed Twitter’s data into a model that accounted for the amount of amplification in each country, political parties’ vote shares in the most recent elections and whether they were in government. Yet even after making these adjustments, the algorithm still favoured conservative parties.
At an individual level, some politicians were amplified by upto 400%, while for some it was below 0 - meaning that content appeared less on algorithmic timelines than it did on reverse-chronological ones. But there wasn't a significant correlation between party affiliation and amplification.
Amplification of far-left and far-right parties was lower than the more-mainstream ones. As a counterpoint, though, we need to account for an apparent trend among the more mainstream parties of shifting their discourse/regulatory agenda towards extremities.
There was no significant difference between when a party was in power or not.
The second part of the study was based on news outlets with significant audiences in the US, since content posted by these politicians accounted for a "small portion of political content on the platform".
(There were no numbers regarding what percentage of the total content on twitter is classified as political, nor what percentage of political content, as classified by Twitter, politicians generally accounted for. For reference, Facebook claims ~6% of content in the US is political)
The study relied on Adfontes and AllSides classification of publications from left to right.
As per the paper, where overlaps existed, AdFontes had a tendency to rate publications neutral more than Allsides, especially on the left. Eg. Ad Fontes classifies NYT Opinion and New York Post as Neutral, while AllSides classified them as left and right respectively. There seemed to more alignment when classifying publications on the right.
Aside: See this thread by Becca Lewis on the idea of classification of media outlets on a left-right spectrum. I also posted some notes from a webinar in which she elaborated on this [SochMuch]


Anyway, let's look at what Twitter's study found.
Based Allsides' classification:
Partisan sources are amplified more.
Partisan right sources more amplified than Partisan left
In contrast to Adfontes' classification where:
Relatively low amplification for partisan left
Neutral category amplified less than others.
The Economist seemingly used NewsGuard ratings to conclude that less accurate sources received more amplification (The graphics on their article are more intuitive than Twitter's paper - I haven't posted them all here).
Source: (The Economist)
Just, great.
In 42: Of Algo-Read'ems, (Col)Lapses in Time, Moderating Moderation, I did write about a study by Jack Bandy and Nicholas Diakopoulos, and spoke about this on a recent episode on Takshashila’s All Things Policy.
Research Plug:
If you recall the paper about harms attributed, then here's a short video you can share with others who might be interested. If you don't, well, then watch it.