Of moderation: stacked and loaded, 'all the rage' and middle(of no)ware,
MisDisMal-Information Edition 48
What is this? MisDisMal-Information (Misinformation, Disinformation and Malinformation) aims to track information disorder and the information ecosystem largely from an Indian perspective. It will also look at some global campaigns and research.
What this is not? A fact-check newsletter. There are organisations like Altnews, Boomlive, etc., who already do some great work. It may feature some of their fact-checks periodically.
Welcome to Edition 48 of MisDisMal-Information
In this edition:
Moderation: Stacked and loaded - Recent events of interest from a “content moderation through the stack” perspective
Outrage is “all the rage” - How users learn to express outrage online and the role of out-group animosity
Middle(of no)ware - Critique of middleware from the July edition of the Journal of Democracy
Moderation: Stacked and Loaded
It has been an interesting week for watchers of content moderation through the stack (27 - Content Moderation Stack and 36 - Must-Carry Water and Internet Scores have looked at this subject).


The anonymous tip line website accompanying the Texas bill that bans abortions after 6 weeks had an eventful journey. First, it was kicked off by GoDaddy with a 24-hour notice. It then moved to Digital Ocean but was subsequently kicked off again before landing up at Epik [Jon Brodkin - Ars Technica]. It seems that Epik discontinued services to it as well (tweet).
Then, there’s the saga of OnlyFans flip-flopping over whether to allow adult content or not. I know what you’re thinking - how is this an instance of content moderation in the stack? I also think you’re onto something. It is not so much as content moderation in the tech stack, as much as it is content moderation driven by some part of the stack - in this case, banks and payment processors [Jillian C York - TheGuardian]
Although the company has rescinded the ban, saying that it has “secured assurances necessary” to support its “diverse creator community”, the incident raises the profile of an important issue that has plagued sex workers for many years: the control that the banking industry – and in turn, content platforms – exerts over their ability to not just make a living, but to simply engage in the same sorts of financial transactions as everyone else.
There are also 2 Amazon-related stories.
First, Amazon (shopping) searches were autocompleting queries that began with “iv” with ivermectin related results, which the company said that autocomplete responses are driven by customer activity and that it would “(block) certain autocomplete responses to address these concerns” [Dieter Bohn - TheVerge]
Related: “Recommended Reading: Amazon’s algorithms, conspiracy theories and extremist literature” [Elise Thomas - ISD Global - page 13 is about autocomplete]

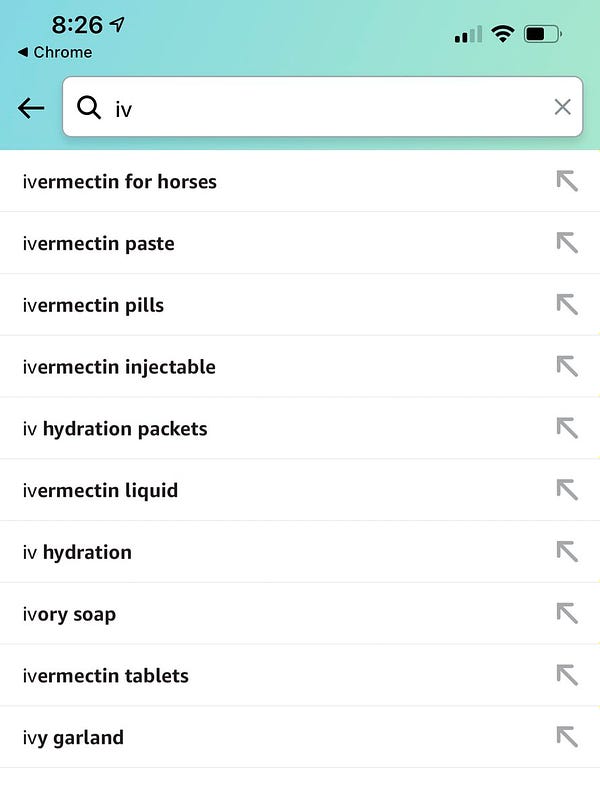
Second, Amazon denied a Reuter’s report that it would ‘proactively’ moderate content on its hosting service [Russell Brandom - TheVerge]
And then, there’s the question about the responsibilities of code repositories in the aftermath of a site that auctioned Muslim women on GitHub [Shephali Bhatt - Livemint]:
.. company inadvertently became an enabler of hate crime recently, but it wasn’t one of the usual suspects like Facebook or Twitter. This time, it was Microsoft-owned GitHub, the world’s largest code repository online
Let’s look at the layers of the content moderation stack as Joan Donovan defined them [CIGIOnline]:
The Amazon autocomplete case is on level 2.
Related: Does Amazon know what it sells? [Benedict Evans]
The OnlyFans case probably belongs here too - unless the framework is expanded to account for the type of downstream moderation of the kind we saw in this case (note: downstream moderation in itself is not unique, in this case, it came from a parallel rather than from up the stream (i.e. higher level).
If AWS and GitHub were to proactively moderate what they host, that would belong to level 3. There’s a case of level 4 too for AWS since its services also include Cloudfront, a CDN.
The Texas website could belong to level 3 or level 5 depending on whether:
‘hosting’ is being considered a subset of cloud services. If not, I’m not sure where it goes in this framework.
They were contracted for DNS only (level 5 only), no DNS (level 3 only), DNS and hosting (level 3 and level 5).
The challenge here is that it is tough to frame consistent rules. But we should expect to see more and more of this as companies that find themselves in lower levels keep relearning that content moderation at scale is impossible to do well. This means pressure on those higher up in the stack will increase. We’ve already seen many cases of this - expect it to become even more frequent as people mobilise and outrage over different types of content/services.

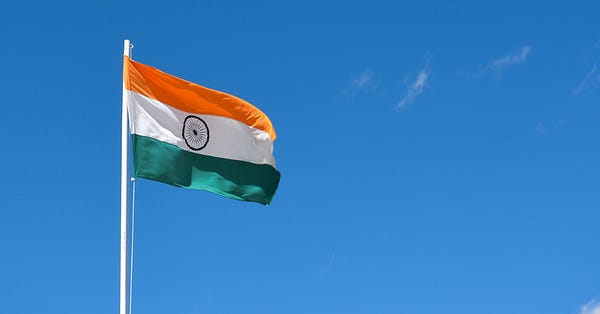
Takshashila is doing a Global Outlook Survey covering domains like India’s bilateral and multilateral engagements, national security concerns, economic diplomacy and attitudes towards the use of force. If this sounds interesting, do click-through to participate.
Outrage is ‘all the rage’
Speaking of outrage, a recent study published by William J. Brady, Killian McLoughlin, Tuan N. Doan, and Molly J. Crockett looked into the very interesting question of how users learn to express ‘moral outrage’ on Digital Communication Networks over time [Science Advances] (46 - Outrage against the machine looked at some aspects of outrage). They conclude:
Past engagement received for outrage expression predicts future outrage expressions (social feedback and reinforcement learning).
Outrage expressions also depend on whether it is frequently expressed in a user’s network or not (norm learning)
Participants in ideologically extreme networks tend to be less affected by past engagement.
They did this through 4 studies.
The first 2 studies relied on datasets that would have contained expressions of moral outrage, but one of which is likely to have less ideologically extreme users. These were to primarily test the reinforcement learning and norm learning hypotheses based on past-behaviour
The next 2 studies involved recruiting participants to a simulated Twitter environment where they were told to learn content preferences in the network (12 tweets) and then given a choice between retweeting a post containing content that was either neutral or expressing outrage and were then shown engagement metrics (30 tweets). The difference between these 2 studies was that in the 2nd one, participants received higher engagement for content that expressed outrage allowing them to study the difference in learning rates based on feedback.
In 46 - I also mentioned a study on the relationship between engagement and out-group animosity. This is a good time to take a closer look at those results.
Related 2: A study by Steve Rathje, Jay J. Van Bavel, and Sander van der Linden on the relationship between out-group animosity and engagement on social media [PNAS]
We report evidence that posts about political opponents are substantially more likely to be shared on social media and that this out-group effect is much stronger than other established predictors of social media sharing, such as emotional language.
For Major Media Outlets
“each additional negative affect word was associated with a 5 to 8% increase in shares and retweets, except in the conservative media Facebook dataset, where it decreased shares by around 2%”
“Positive affect language was consistently associated with a decrease in shares and retweet rates by about 2 to 11% across datasets.”
Liberal
In-group language: On Twitter, increased retweet rates. On Facebook, no effect.
Out-group language: On Twitter and Facebook, strong predictor of engagement. Also, a strong predictor for angry and haha emoji reactions.
Conservative
In-group language: Twitter, increased retweet rates (a little more than liberal dataset). Facebook, increased engagement.
Out-group language: Twitter and Facebook, increased retweet rates/engagement. Also, a strong predictor for angry and haha emoji reactions.
“on average, the angry reaction was the most popular of the six reactions for both liberals and conservatives in the news media accounts,”
For politicians (Congress Members)
Emotional language
Negative language: “consistently increased retweet rate and shares across all datasets by 12 to 45% per negative affect word, with the effect size being largest in the conservative Twitter dataset”
Positive language: “slightly decreased shares by roughly 2 to 5%, except in the conservative Twitter accounts”
Moral-emotional language: “consistent positive effect across all datasets, increasing retweets and shares by roughly 5 to 10%”
Liberal
In-group language: “political in-group language decreased retweet rate on … and only slightly increased shares on Facebook”
Out-group language: “very large predictor of retweets in the liberal congressional Twitter … and of shares in the liberal congressional Facebook accounts”
Conservative
In-group language: “political in-group language decreased retweet rate on Twitter … and slightly increased shares on Facebook”
Out-group language: Same pattern as Liberal Congressional members.
For both sets: “Posts about the out-group strongly predicted negative reactions, such as “angry” reactions”. And, “posts about the political in-group predicted “love” reactions”.
Across all 8 datasets
“each political out-group word increased the odds of a retweet or share by about 67%”, while “in-group language, on the other hand, did not have a statistically significant effect on shares and retweets”.
“Negative affect language increased diffusion by about 14% per word”, moral-emotional language by 10% per word, and positive affect language “decreased diffusion by about 5% per word”
Middle(of no)ware
Now, I’ve written about middleware in 44 - Unbundling Social Media.
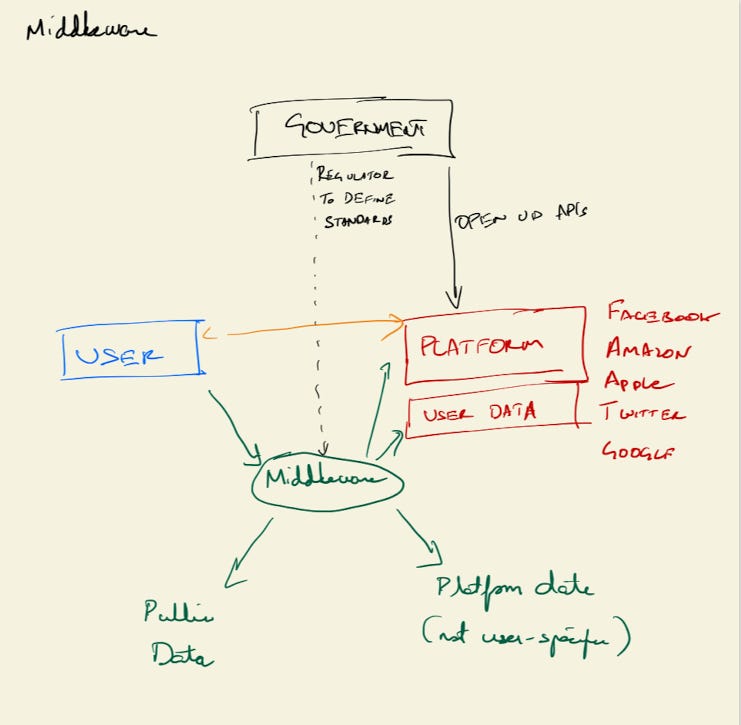
The July edition of the Journal of Democracy included a special section titled ‘The Future of Platform Power’ focused on middleware which includes some interesting critique of the approach.
Daphne Keller (who proposed Magic APIs and is optimistic about middleware) poses four questions that need to be addressed:
Quality of service: Can middleware companies provide an equivalent or superior experience compared to the incumbents, and can they process the same volumes of data?
Business models: How will middleware companies make profits? What incentives do platforms have to share revenues?
Curation Costs: Large DCN firms employ/contract a significant number of people in content moderation roles. How can the ‘solved’ aspects of content moderation be replicated so that they can focus on the unique/un-solved aspects?
Privacy: Are data generated by interactions in a users’ network available to middleware companies? If yes, there are privacy implications. If no, it limits the utility of middleware solutions and, therefore, their ability to compete with incumbents.
Joan Donovan and Robert Faris believe middleware is ‘fragmentation by design’ and question whether it will be lead to outcomes significantly different from the current system. They also raise the concern that middleware could, in theory, exacerbate polarisation. These are recurrent themes in most criticism of the approach. They summarise the critique of the status quo from the (American) political left and right.
Critique of status quo from the political left:
“Platforms not doing enough to root out extremism, online abuse and disinformation”.
Argument in favour of greater platform action: “the openness of the internet enables malicious actors to build power” (disinformation, social division, threatening opponents into silence, and that tech companies have a responsibility to address harms perpetrated on their platforms).
Concerns that operating disinformation campaigns is politically convenient + practical, and profitable.
Critique of status quo from the political right:
Disproportionately target conservative voices, i.e. “content moderation going too far.”
Believe that the actions of platforms are “motivated solely by partisanship”.
Critique of middleware
Make the claim that Fukuyama's diagnosis is too narrow, overlooks motivated bad actors, US political right that has adopted anti-democratic positions and governments around the world exercising authoritarian impulses. “More technology cannot solve the problem of misinformation-at-scale”
Claim that Fukuyama sides with conservatives in arguing that it is "neither normatively acceptable nor practically possible to prevent them from expressing opinions to this effect. For better or worse, people holding such views need to be persuaded, and not simply suppressed.”
That it is a “renewed argument for the marketplace of ideas”, and the internet has demonstrated that more speech has not been a remedy for bad speech.
Dipyan Ghosh and Ramesh Srinivasan, like Donovan and Faris, believe the current set of challenges go beyond the narrow, content moderation-focused approach of middleware. Nathalie Maréchal raises the absence of a business model as a red flag:
This is essential: Middleware firms will have their own set of incentives and will need to be accountable to someone, be it a board of directors, shareholders, or some other entity. Incentives and accountability both depend on how the “middleware” providers will make money.
She argues that middleware simply:
displaces the perverse incentives inherent to the business model (targeted-advertising.
In a response essay, Francis Fukuyama states:
Our working group’s promotion of middleware rests on a normative view about the continuing importance of freedom of speech. Middleware is the most politically realistic way forward.